1. Research background and status quo
1.1 Research background
With the rapid development of transportation worldwide, reliable and accurate vehicle positioning is realized in all kinds of vehicles. Navigation and safety-related applications such as route navigation, autonomous driving and intelligent transportation are becoming increasingly important.
Global Navigation satellite systems (GNSS) such as the Global Positioning System (GPS) can provide vehicles with accurate absolute position information has become the most commonly used technology in vehicle positioning system. Generally speaking, GPS can provide accurate position, speed and time data, but it does not provide attitude information. The data rate is mostly below 10Hz, which means that GPS cannot sense dynamic changes fast enough in some applications. Moreover, because it needs to receive at least four satellite signals, satellite signals are easily blocked in cities, canyons, underground, tunnels and other places and cannot provide navigation services. At the same time, GNSS is vulnerable to deception and interference in the environment of cities and dense forests, which leads to the decline of navigation accuracy. In addition, it is not reliable in military applications.
INS (Inertial Navigation System) is a kind of autonomous navigation system. Be susceptible to external influences. It can output not only position and speed, but also attitude information, and the data rate can reach 100Hz or even higher. INS uses the measurement values of inertial measurement unit for navigation calculation. IMU usually consists of a pair of mutually orthogonal triaxial gyroscope and triaxial accelerometer, which respectively measure the angular velocity and acceleration of the carrier relative to the inertial coordinate system, and output the relative position of the carrier through coordinate system conversion and integration. The resulting problem is that sensor errors such as scale factor errors, non-orthogonal errors and random noise will also accumulate with integration.
These features of GNSS and INS make the integration of GPS and INS widely studied. Kalman Filtering (KF) is a classical data fusion method. INS/GPS integrated navigation has been successfully implemented in different integration strategies (loose combination, tight combination, and deep combination). In addition, Jollier proposed untraceless Kalman filter (UKF), which uses untraceless transform (UT) to obtain statistical properties of states without linearization processing. Shin applied untracked Kalman filter and extended Kalman filter (EKF) to low cost MEMS INS/GPS integration. Sasiadek and Loebis proposed an adaptive scheme to adjust Kalman gain according to the residual difference between INS and GPS. These methods have improved the accuracy of the navigation system to varying degrees. However, navigation errors will still accumulate when GNSS loses signals.
Expensive high precision inertial sensors have excellent error characteristics and can be long after the loss of GNSS signal. Time maintains the accuracy of navigation results, but it is difficult to be widely used in vehicle navigation due to its high cost, large volume and weight. With the rapid development of semiconductor technology, inertial sensors based on micro-electro-mechanical systems emerge as The Times require, which can be integrated on silicon wafers and has the advantages of low cost, small size, light weight and low power consumption, but its disadvantages are also very obvious, because the sensor error is very large. When there is no GPS signal, the error of navigation system will diverge quickly. It is urgent to solve this problem.
1.2 Research status at home and abroad
When the GNSS system is disturbed or in areas such as cities, canyons, jungles, etc., GNSS navigation accuracy is rapid
To reduce or even completely lose navigation ability, it is imperative to study independent navigation system. In order to reduce the error drift of inertial navigation system, researchers put forward many methods, including artificial intelligence, vehicle dynamics constraints, auxiliary sensors, frequency domain denoising algorithm, rotation modulation technology, etc.
With the rapid development of artificial intelligence, some scholars have developed navigation algorithms based on artificial intelligence, such as nerve
Neural Network (NN), Support Vector Regression (SVR), fuzzy logic, etc. The basic idea of artificial intelligence is to achieve nonlinear mapping of input to output through training. Relationships between inputs and outputs can be established without knowing exactly what the model of the system is. Therefore, more and more scholars apply neural network method to INS/GNSS integrated navigation information fusion to predict and correct INS navigation parameters when GNSS signals are interrupted. Sharaf uses radial basis (RBF) neural network to predict the position and velocity errors of INS in the absence of GNSS signals, thus improving the positioning accuracy when GPS signals are lost. BP neural network is used to update position and speed, and experimental results show that it is superior to Kalman filter in navigation accuracy. However, these studies did not consider the correlation between errors and past information. A. Noureldin et al proposed that Input Delay Neural Network (IDNN) fully consider the dependence of INS errors on time. The position and speed errors of INS were modeled based on some current and past INS position and speed data, so as to simulate the error trend of INS and provide stable navigation error estimation. By comparing several methods, Kamal Saadeddin found that the optimal performance can be obtained by taking position and speed as input in the time-delay neural network.
However, navigation algorithms based on artificial intelligence require a lot of training data to ensure the accuracy of the prediction.
Zero Velocity Update (ZUPT) is the most commonly used vehicle motion constraint model, but only can be used when the vehicle is stationary. Dissanayake applied Non-holonomic Constraint (NHC) to assist navigation, which assumes that the vehicle tire never leaves the ground and that the skyward and lateral velocities are zero so as to estimate the vehicle roll angle, pitch angle and forward velocity. Ojeda and Borenstein used the complementary motion detection characteristics of accelerometer and gyroscope to estimate attitude. The basic idea is to update attitude by using the attitude angle derived from the accelerometer when the vehicle is stationary or in uniform linear motion. Hamad Ahmed modeled the external acceleration of the vehicle as a low-pass process, and used the accelerometer for attitude estimation when the vehicle was not at rest or at uniform speed.
Auxiliary sensors include magnetometer, odometer, vision sensor, etc. The odometer can provide speed and the strong correlation between position information, velocity and sensor error enables sensor error to be observed in the filter.
However, the accuracy of the vehicle's own odometer is low, and the data transmission rate is different and difficult to apply. With the advance of electronic technology and manufacturing technology, MEMs-based magnetometer can provide absolute heading information to assist inertial navigation system. However, in practical applications, there is usually a local magnetic field, making the measurement results of the magnetometer inaccurate. With the development of image recognition technology, visual navigation is emerging gradually. Visual aided integrated navigation system has attracted a large number of scholars to study, but its disadvantage is that it is relatively expensive and can not be used at night.
The random error of inertial sensor can be divided into high frequency component and low frequency component according to frequency. High frequency component has white noise characteristics, while low-frequency component has correlated noise characteristics. One way to deal with high frequency noise is to use wavelet decomposition to denoise measurement data of inertial sensor. Skaloud uses wavelet decomposition algorithm to remove high frequency noise of IMU data, which successfully improves positioning accuracy. However, it requires prior information on bandwidth of real motion dynamics of typical land vehicles and spectral characteristics of wavelet denoising algorithm. It is difficult to apply. In addition, MEMS IMU still has large noise due to the serious overlap between zero-bias instability noise and signal frequency band.
The concept of rotary modulation technology (RMT) originated from the platform inertial Navigation system (PINS) in the 1960s. It was proved by E. S. Geller that platform rotation can reduce the position error caused by sensor noise. With the development of technology, SINS gradually became the main trend of INS. In 1980, a scholar fixed IMU on a turntable and studied the implementation of rotary modulation technology in SINS. After the IMU rotated around the rotation axis for a complete cycle, The static deviation of the non-rotational axis will be adjusted into a periodic signal with zero mean value, and the deviation will no longer accumulate over time, thus reducing the navigation error, but the error on the rotational axis is not eliminated. In addition, when IMU rotates, scale factor error will lead to additional error on the rotation axis, while non-orthogonal error will lead to additional error on the non-rotation axis. Shuang Du's simulation and experiment on rotational modulation of MEMS IMU indicate that rotational modulation can significantly improve the observability of the system. Thus, the positioning accuracy of MEMS IMU is greatly improved. The research of Jussi Collin shows that rotation can eliminate velocity random walk noise and zero-bias instability noise, but cannot eliminate white noise.
Traditional RMT applications require a dedicated turntable, which requires additional control systems and motors, it violates the original intention of MEMS sensor application: low cost, low weight, low power consumption and small size. However, for vehicle applications, the wheel itself is a turntable. If the rotation Angle of the wheel can be accurately obtained, the rotation of the wheel can be used to replace the special turntable to reduce the inertial navigation error.
Ericco not only has FOG IMU but also MEMS IMU, high-precision navigation level and north-seeking level. If you are interested , please feel free to contact us.
More Technical Questions
1.Application of IMU in UAV Flight Control System
2.What are the advantages of MEMS IMU?
3.Internal Structure Analysis of IMU
4.Calibrating Processing of MEMS IMU ER-MIMU-02
Products in Article
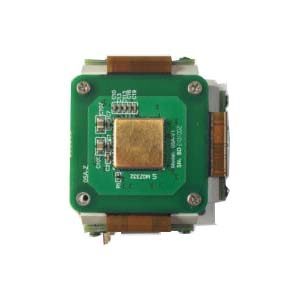
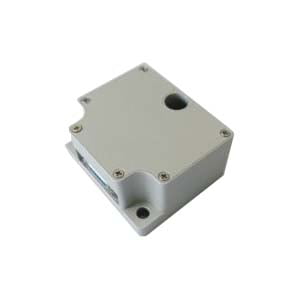
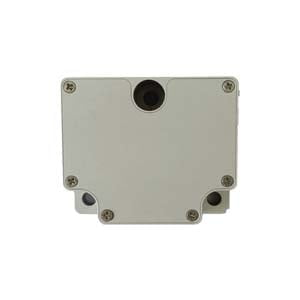
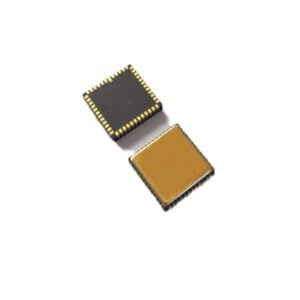
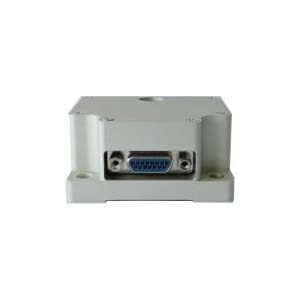
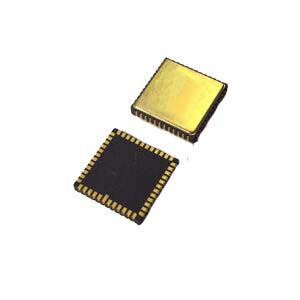