North seekers generally use gyroscopes and accelerometers to statically seek north through a multi-position method. Errors in gyroscopes and accelerometers can be divided into deterministic errors and random errors. Deterministic errors can be accurately measured and compensated through multi-position transposition, while random errors have become an important factor affecting the accuracy of north seeking. To this end, various methods have been used to model and filter this random error.
In terms of gyro random error modeling, some experts use the auto-regressive moving average model (ARMA(n,m)) to establish a random error model for fiber optic gyroscopes (FOG). Some also use wavelet transform to model and filter FOG random errors, and Allan variance analysis method and its improved methods can be used to analyze various noises in the data before and after modeling and filtering.
This paper takes the north seeker using FOG and accelerometer as the core components as an example. On the basis of the above, the improved second-order autoregressive model-AR(2) model was used to carry out online modeling and filtering research on the measurement signal in the gyro north seeker, which improved the north seeking accuracy of the gyro north seeker.
The ARMA(n,m) model requires that the signal must be stationary, normally distributed, and have a zero-mean time series. Theoretical derivation and experiments prove that the static output data of FOG and accelerometer can basically be regarded as stationary and normal time series. However, due to the existence of ground speed and FOG bias as well as gravity acceleration and accelerometer bias, the static output data of FOG and accelerometer do not meet the condition of zero mean. In order to realize online modeling of the static output signals of FOG and accelerometers and real-time filtering of random errors, this article uses an improved ARMA(n,m) model.
Although the AR(2) model is not the best model for the accelerometer random error, considering the relatively small model error of the AR(2), the consistency of the FOG and accelerometer model errors, and calculation amount of the Kalman filter of north seeker, In this paper, the random error model of FOG and accelerometer is uniformly selected as the improved second-order auto-regressive model-AR(2) model. Its online construction and real-time filtering process are as follows:
Assume that the stationary, normal and zero-mean time series are x1, x2, x3,...xk-2, xk-1, xk,..., then the AR(2) model can be expressed as:
Let the static output data of FOG and accelerometer be y1, y2, y3,…yk-2, yk-1, yk,…. This series can be regarded as a stationary and normal time series. Only after zero-mean processing can the modeling by equation (1) be applied. Since the ideal values of the static output data of FOG and accelerometer are constants, their average value can be set as y, and the stationary, normal, zero-mean time series can be expressed as:
Substitute formula (2) into formula (1) to get:
Since the average value y of the static output data of the FOG and accelerometer can be regarded as a constant, the model parameters 1 and 2 to be estimated are also fixed numbers, so the following constants can be set:
Then equation (3) becomes
Equation (4) is the improved second-order autoregressive model-AR(2) model of the static output data of FOG and accelerometer. Based on the time series of the static output data of the FOG and accelerometer, the model parameters 1 and 2 and the constant c can be estimated in real time by the least square method, so that the model can be determined online in order to filter the random errors of the static output data of the FOG and accelerometer in north finder.
In short, as an emerging inertial product, north seeker is very popular in the military and civilian fields. Whether it is drilling, mining or satellite navigation, there is huge market for development.As inertial technology is penetrating into our lives, we have a higher level of pursuit for the quality and level of inertial devices. With our technical support, we continue to carry out multi-position north finding program in the north seeker product series ER-FNS-01,ER-FNS-03, improve the precision of gyro and accelerometer, establish static model and other methods to reduce temperature drift and eliminate errors, if you are interested, you can learn more parameter information on the website.
More Technical Questions
1.Research and use of north finder for mining drilling rigs
2.System Analysis of MEMS North Seeker
3.How to Process the Data of FOG North Seeker
4.Research on Anti-environmental Interference of North Finder
5.Analysis and Calibration of North Seeker Transposition Error
6.Analyze the Software Design of North Finder
Products in Article
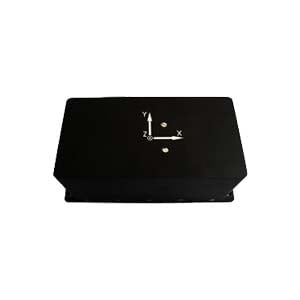
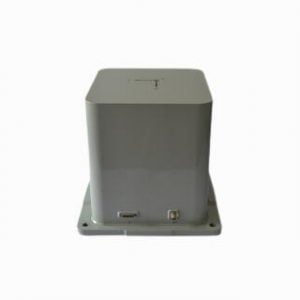
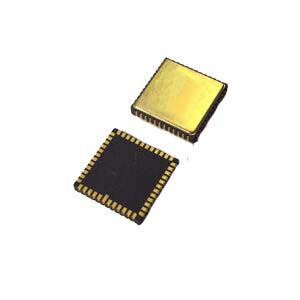
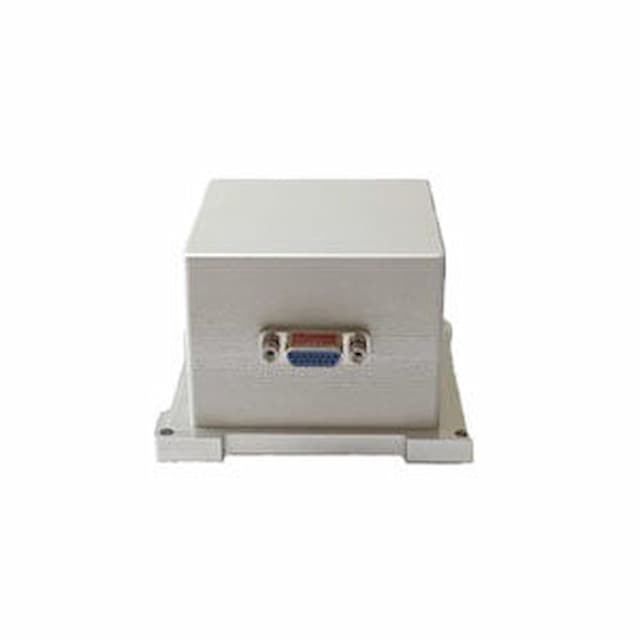
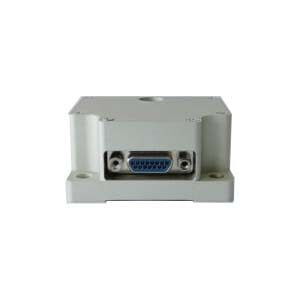
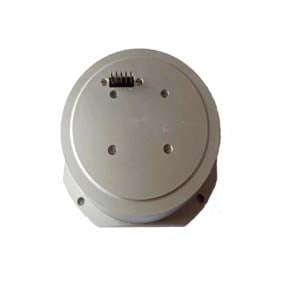